Biology goes digital - data, processing and AI as enablers of a new kind of medicine
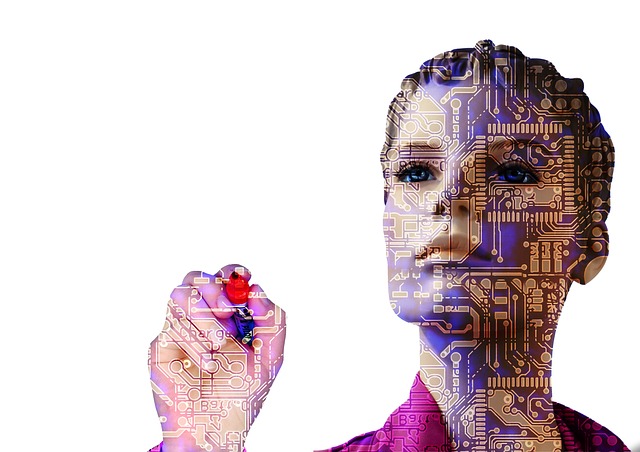
Computing makes leveraging the convergence of exponential technologies in healthcare possible. The major drivers are the increase in the memory capacity and processing power and the improvement of algorithms. It serves as the connecting piece between the exponential increase in data and its potential use via the exponential decrease in cost and difficulty of biologic applications through e.g. gene editing.
This is part 4 of my series about exponential technologies in healthcare. In the previous part, I’m looking at the gene editing technology CRISPR. Read in part 2 how the exponential development in genome sequencing has resulted in an explosion of genome data. The series intro puts all of it into context and explains my hypothesis that the convergence of several exponential technologies in healthcare represents the largest opportunity of our time.
Truly Big Data
In healthcare vast amounts of data are generated due to genome sequencing and the application of sensors.
The human genome consists of 3 billion base pairs. It contains about 22.000 coding genes.
The size of the whole sequenced genome is around 200 GB. As more and more people, animals, plants and bacteria get sequenced, it is expected that genomic data will outpace all other data sources in terms of size.
At the same time, increasingly larger parts our lives can be captured digitally. Sensors in our phone can constantly monitor our behaviour. The things we post on social media complement this data.
We’re speaking of big data in the true 4Vs way that needs to be stored and analysed in an efficient manner to become valuable. The real value lies in combining biological data on the different codes of live with longitudinal behaviour data. This is only possible through the connecting piece of computing.
Precision medicine in oncology provides a good example of how this is already being applied. However, I believe that probably an even larger opportunity lies in chronic disease care and wellness.
Real Life Applications
The simple idea behind precision medicine is that we’re different in our genetic code. Therefore, our response to drugs will differ. The goal is to find the right drug, for the right patient, at the right time, every time.
How is this supposed to work?
The first step is to establish the genetic fingerprint of a tumor in order to know what traits it expresses. This provides important information on what to target therapeutic efforts on. Shall we rather disable its way to grow blood vessels or disrupt one of its signaling chains?
Cancer can be caused by a mutation in any of the 3b base pairs. This makes more combinations possible than stars in the sky. Although some will appear more often than others, we need to cover the rare ones as well, in order to really understand this disease. No human doctor alone would ever be able to account for all combinations.
The next question is what the best therapy is. Ideally we wouldn’t only consider cancer treatments, but look at all known therapies and establish connections with the drivers of the tumor.
The question really becomes, what is the right therapy for the right patient at the right time?
The right time component is relevant, because cancer can develop and adapt to a therapy. The approach right after diagnosis will potentially be a different one when compared to the 3rd line therapy.
Precision Medicine Infographic from Dana-Farber/Boston Children’s
AI
Understanding cancer and picking the right therapy is impossible without using modern algorithms and ubiquitous computing power. Tracking disease progression based on the therapy chosen, given a certain fingerprint of the tumor allows us to generate training data. This data can be used in order to match cause (therapy) and effect (progression) given driving parameters (molecular fingerprint) and train a model.
When applied on a large scale, a system will be able to learn the driving factors and recommend therapies that are most likely to delay the disease progression. In the process AI will also help us discover new methods to address cancer in ways that we haven’t even thought before.
Just like AlphaGo used moves that nobody has ever thought of before thereby providing the grandmasters a new understanding of their own game, will AI provide doctors and molecular biologists with new insights into oncology.
Data heavy applications such as genomics are good candidates for machine learning applications. Leveraging the ubiquitous computing power available and combining different data sets (e.g. genome, sensors), machine learning can finally be taken advantage of in biotech.
NGS provides us with data on how different levels of the biologic systems look like. While the gene is the first order of being, there are several levels that form a complex system over RNA, proteins and others, such as the microbiome.
AI will finally allow us to combine all the levels of the biologic system and help us answer question such as: How can knowing your genes help you pick the perfect drug? How can knowing your microbiome help you pick the perfect diet? How can we prevent depression by knowing your proteome?
These kinds of complex recommendations that are tailored to you, are only possible with the help of machine learning.